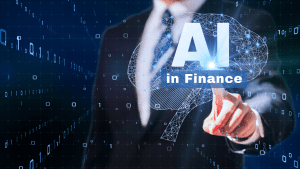
In today’s rapidly evolving financial landscape, artificial intelligence (AI) has emerged as a powerful force reshaping how financial institutions operate and deliver services. From automated customer service to sophisticated fraud detection systems, AI technologies are revolutionising every aspect of the financial sector.
What is artificial intelligence (AI) in finance?
Artificial intelligence in finance refers to the application of machine learning algorithms, natural language processing, and data analytics to enhance financial services and decision-making processes. This integration allows institutions to automate complex tasks, analyze vast amounts of data in real-time, and improve overall operational efficiency.
AI systems can independently learn and adapt by processing historical data and identifying patterns. In finance, this capability enables institutions to make more informed decisions about risk assessment, investment strategies, credit scoring, and customer interactions. Moreover, AI technologies can simulate various market conditions and predict future trends, giving financial professionals critical insights to enhance their strategies.
One key component of AI in finance is the utilization of big data. Financial institutions have access to an immense volume of data generated from transactions, customer interactions, market movements, and more. AI algorithms can sift through this data, extracting meaningful insights that help in recognizing risks, forecasting market behavior, and personalizing customer experiences.
In summary, AI in finance represents a significant shift towards data-driven decision-making, allowing financial institutions to operate with greater accuracy, efficiency, and customer focus. As these technologies continue to develop, their influence is expected to grow even further, setting the stage for a smarter, more responsive financial ecosystem.
The Financial Industry’s Pain Points
Inefficiency and High Operational Costs
Traditional financial institutions have long struggled with manual processes that are not only time-consuming but also prone to human error. Back-office operations, document processing, and compliance checks often require significant human resources, leading to high operational costs that ultimately impact customer pricing.
Rising Fraud and Security Threats
As digital transactions increase, so do the sophistication and frequency of fraud attempts. Financial institutions face enormous challenges in detecting and preventing fraudulent activities while maintaining seamless customer experiences.
Customer Expectations Gap
Modern consumers expect personalized, instant, and 24/7 financial services. Traditional banking hours, generic financial products, and slow processing times no longer meet these expectations, creating a significant gap between what customers want and what many institutions provide.
Regulatory Compliance Burden
Financial institutions must navigate an increasingly complex regulatory environment. Compliance failures can result in substantial penalties, but maintaining compliance manually requires significant resources and still risks human oversight errors.
Investment Decision Complexity
In investment management, analyzing vast amounts of market data, identifying patterns, and making timely decisions has become increasingly difficult for human analysts alone, potentially leading to missed opportunities or suboptimal portfolio performance.
How AI is Transforming Financial Services
Banking Revolution
Intelligent Automation of Routine Tasks
AI-powered robotic process automation (RPA) is streamlining back-office operations by automating repetitive tasks like data entry, document verification, and transaction processing. JPMorgan Chase’s COIN (Contract Intelligence) platform exemplifies this transformation, reviewing commercial loan agreements in seconds rather than the 360,000 hours previously required by lawyers and loan officers annually.
Enhanced Customer Experience
Conversational AI and chatbots now handle routine customer inquiries, providing instant responses regardless of time or day. Bank of America’s virtual assistant, Erica, serves over 19 million users, handling everything from transaction searches to bill payment reminders, significantly reducing call center volume.
Advanced Fraud Detection
Machine learning algorithms analyze transaction patterns in real-time, identifying suspicious activities with unprecedented accuracy. These systems can detect anomalies that human analysts might miss, reducing false positives while catching more actual fraud attempts.
Insurance Transformation
Automated Claims Processing
AI systems are revolutionizing claims management by automating assessment and processing. Lemonade, an insurtech company, uses AI to process simple claims in seconds rather than days or weeks, dramatically improving customer satisfaction.
Risk Assessment and Pricing Precision
Machine learning models analyze vast datasets to assess risk more accurately, enabling more personalized pricing. This precision allows insurers to offer competitive rates to low-risk customers while appropriately pricing coverage for higher-risk scenarios.
Predictive Maintenance and Loss Prevention
In property insurance, AI-powered predictive analytics help identify potential issues before they cause damage. Some insurers now offer IoT-connected home monitoring systems that can detect water leaks, electrical problems, or security breaches, preventing costly claims.
How does JP Morgan use AI?
Predictive Maintenance and Loss Prevention
Harnessing the power of advanced analytics and artificial intelligence, firms like JP Morgan are not only transforming customer engagement and risk management but are also implementing predictive maintenance strategies to identify potential operational disruptions before they occur. By leveraging AI’s capabilities, JP Morgan can enhance its infrastructure reliability, ultimately leading to smoother banking operations and improved customer satisfaction.
Infrastructure Monitoring
JP Morgan employs AI algorithms to monitor the health of its IT infrastructure continuously. Real-time analytics allow the firm to track server performance, application response times, and network traffic patterns. By predicting potential failures, the bank can proactively address issues, such as server outages or connectivity problems, minimizing disruptions that could hinder customer transactions or data access.
Financial Asset Management
In addition to IT maintenance, AI plays a crucial role in overseeing financial assets. By predicting asset behavior based on historical data and market trends, JP Morgan can optimize the portfolio management process. This predictive modeling allows for better investment strategies, ensuring that financial assets are essentially maintained at their peak value while minimizing unnecessary risks that could lead to losses.
Fraud Prevention as a Preventive Measure
Beyond maintenance and operational efficiency, AI’s application in fraud prevention exemplifies predictive capabilities. JP Morgan utilizes machine learning to analyze transaction data
Investment Management Innovation
Algorithmic Trading and Portfolio Management
AI systems analyze market conditions, historical patterns, and news in milliseconds to execute trades at optimal times. Quantitative hedge funds like Renaissance Technologies leverage these capabilities to generate consistent returns regardless of market conditions.
Personalized Financial Advice
Robo-advisors use AI to create and manage investment portfolios tailored to individual goals, risk tolerance, and time horizons at a fraction of traditional management fees. Platforms like Wealthfront and Betterment have democratized access to sophisticated investment strategies previously available only to wealthy clients.
Alternative Data Analysis
AI enables the analysis of non-traditional data sources such as satellite imagery, social media sentiment, and web traffic to inform investment decisions. Hedge funds increasingly use these alternative data points to gain competitive edges in market prediction.
Challenges and Considerations
Data Privacy and Security Concerns
The implementation of AI requires vast amounts of sensitive financial data, raising significant privacy concerns. Financial institutions must balance innovation with robust data protection measures to maintain customer trust.
Algorithmic Bias and Fairness
AI systems can inadvertently perpetuate or amplify existing biases in lending, insurance, or investment decisions. Organizations must implement rigorous testing and oversight to ensure AI-driven decisions remain fair and unbiased.
Regulatory Compliance
As AI applications in finance evolve, regulators are working to develop appropriate frameworks. Financial institutions must navigate this emerging regulatory landscape while ensuring their AI systems maintain compliance with existing regulations.
Human-AI Collaboration
The most successful implementations of AI in finance complement rather than replace human expertise. Finding the right balance between automation and human judgment remains a critical challenge for financial institutions.
The Future of AI in Finance
Quantum Computing Integration
As quantum computing matures, financial institutions will gain unprecedented computational power for complex risk modeling, portfolio optimization, and fraud detection, potentially revolutionizing financial analysis capabilities.
Decentralized Finance (DeFi) and AI
The combination of blockchain technology and AI promises to create more transparent, efficient financial systems with reduced intermediaries and enhanced security protocols.
Hyper-Personalization
Advanced AI will enable truly personalized financial services tailored to individual life events, spending patterns, and financial goals, creating “financial companions” rather than just service providers.
Expanded Financial Inclusion
AI-powered alternative credit scoring and simplified interfaces will help bring financial services to previously underserved populations, potentially reducing global financial inequality.
Conclusion
Artificial intelligence is not merely enhancing existing financial services but fundamentally transforming how financial institutions operate. From improving operational efficiency and detecting fraud to delivering personalized customer experiences and optimizing investment strategies, AI technologies are addressing long-standing pain points throughout the financial sector.
While challenges remain, particularly around data privacy, algorithmic fairness, and regulatory compliance, the trajectory is clear: AI will continue to drive innovation across banking, insurance, and investment services. Financial institutions that successfully integrate AI capabilities while maintaining human expertise will be best positioned to thrive in this new landscape.
For consumers, businesses, and investors alike, the AI revolution in finance promises more accessible, efficient, and personalized financial services than ever before. The question is no longer whether AI will transform finance, but how quickly and completely this transformation will unfold.